CRISPR Unedited featuring Bernhard Schmierer (Karolinska Institutet)
This is a machine transcription and therefore it may contain inaccuracies, errors, or mispronunciations. Notice an error you think needs changing? Please contact the Bitesize Bio team using this form: https://bit.ly/bsbtranscriptions
Welcome to CRISPR Unedited,
a bite-sized bio podcast hosted by Anton Adamson.
Today on CRISPR Unedited, we chat to Bernard Schmear,
who heads the CRISPR Functional Genomics facility at the Carol Green Street in
Sweden. We learn about the vast number of cells required for CRISPR screens.
This means, uh, 500 cells to a thousand cells per guide.
So you are handling roughly 80 million cells,
Hear about must read literature on Christmas greens.
Read the Review by John Dench. That's called, am I Ready for crispr?
I think we also call it, uh, gospel according to John.
And we discover what the future holds.
We are looking a lot into, uh, base editing screens,
uh, where you can now,
instead of knocking out an entire gene or activating an entire gene,
you can now actually introduce point mutations,
All this and more in this episode of Crisp Bur Unedited.
Hello everyone, and welcome to this podcast from Bite-Size Bio.
My name is Anthony sson,
and I run a core facility called the Genome Editing Unit,
where we use CRISPR Cas nine to engineer, uh, cultured cells, uh,
genetic modified mouse models and, and modified flies as well. Now,
my facility focuses on one gene at a time. Um, we might use CRISPR to say,
knock out that gene of interest in a cell line and explore its function
afterwards. Maybe put some mutations in that, uh, same gene or tag it with, uh,
a fluorescent protein. But today's podcast guest, um,
he doesn't concern himself with one gene at a time.
He operates at scale tags in the entire genome and knocking out every single
gene using the so-called CRISPR knockout screens. So,
I'd like to welcome Bernard, uh, schmear to the podcast. Welcome, Bernard.
Thank you, Anthony.
Uh, so to start with, maybe we could get an idea of your background.
Tell us a little bit about yourself, uh,
how you came to work in the field of performing Christmas greens.
Yeah, sure. Um, so I am, I'm originally from Austria. I did my, uh,
undergrad in biochemistry and my PhD at Vienna University. Um,
and then moved on to London to do a postdoc, uh,
to Cancer Research uk, London Research Institute. So,
cancer Research UK had actually just been founded a year prior to,
to me joining that was in 2003, I think. Uh,
spent five years there working on T g PTA signaling mechanisms of TG F pta,
signal transduction from the membrane to the nucleus.
And while doing this,
I slid a little into mathematical modeling of dynamic processes,
and I pursued this, uh, by moving to Oxford, uh,
where I did another postdoc with, uh, be Novak at, uh,
department of Biochemistry in Oxford. Um,
and since 2012, I have been in Stockholm.
I came here initially to join as a senior scientist, the lab of uc,
who is now also professor in Cambridge, actually. Um,
and screening. Yeah. Um, I was,
I was at the time supposed to run a project, uh,
where we were looking for genes affecting, uh, cell size regulation,
and we were doing this gene trapping that had been published in 2011, I think.
Uh, but then immediately when, uh,
the first Christmas screening papers came out in, in early 2014,
we jumped on that bandwagon. And this was my start on crispr. Um,
the cell size bit never flew,
so never anything came out of that. Um, but yeah,
I think I learned the, the CRISPR screening pretty from pretty early on.
And then we did a lot of screens with transcription factor libraries on, uh,
different, uh, cancer cell types. So it was essentially like, uh,
a little bit of what, what depth map is now doing, of course, at,
at a huge scale genome-wide with a lot of cells.
And since 2017,
I'm running a facility that's now called CRISPR Functional Genomics.
We also do the precision editing just as you do, um, with varying success.
Um, but we also, and our main focus actually is on, is on the,
on the transcriptomic, sorry, on the, on the functional genomics. So really, I,
I I tend to call it functional genomics, CRISPR based functional genomics,
because it's not just knockout screens, but we might get to that.
Yeah, absolutely. Um, so like I say, we,
we tend to just focus on knocking out a single gene at time,
and we haven't performed any knockout screens at all. Um,
we are looking into it. We've got a number of people locally who are re uh,
interested in applying this technology and their research. And from the outset,
you know, as an outsider looking in, it looks quite daunting, you know,
from what I can tell, there's a hell of a lot of cell culture involved. So,
you know, how do you manage this?
Yeah, so there are in principle two ways of doing this, right? Uh,
there is an array, uh,
there are array screens where you have each guide against each chain in one well
of a microt title plate. Um, this is not what we do at all. So, um,
as you were alluding to, we are doing pool screens, and for pool screens,
as you say, you need quite a lot of cells.
So for the genome-wide libraries that are around now and most commonly used,
they contain about 80,000 guides. So four guides per gene.
And in order to keep the library coverage through a screen,
one commonly run the screen at a 500 x to a thousand x, this means,
uh, 500 sales to a thousand sales per guide.
So you are handling roughly 80 million sales,
maybe 40 million minimally. Um,
that can be quite daunting. Uh, one gets used to it.
So I don't think it's the biggest issue really, but, uh,
you should not be scared of having to handle like, uh,
around 80 T 1 75 large cell culture flasks at a time.
Um, yes, it also needs quite some incubator space sometimes if so,
running at the same time. Um, but that's okay.
Um, I think it's one of the, it's, it was one of the things you get used to, um,
people find it quite scary in the beginning,
but you get used to it fairly quickly.
So how, how many members of staff do you have then?
Does I assume it takes quite a few people to room with these screens if there's
all this cell culture involved?
Yeah, so we currently, we are six altogether, so including myself,
I'm not in the lab ever, which is probably for the better. Um,
but, uh, five people, no, sorry,
four people actually work on the Christmas screens. Um, mainly.
So we also do a lot of tech development and other things.
So it is not just Christmas screens,
and I have one person doing the precision genome editing focusing full-time on
that, and the others help her out by commonly with that.
Um, so it works very well. It is not so much limited by people.
It can be limited by, as I said, like incubator space or you have to, or virus,
virus room space and these kind of things. Uh,
so you cannot run too many screens at the same time. It has to be one at a time.
Just one a time. Yeah. Yeah.
Yeah.
And how long would each screen typically take?
Um, yeah, um,
brings me maybe to another challenge of the screen. So you,
you normally do the screen such that you create a S nine expressing cell line
first, uh,
and creating a S nine expressing cell line that actually stably maintains S nine
expression can be quite challenging. Um,
so we do that by having a P F P on the CS nine.
And we sort multiple times often over several weeks,
multiple times until we get a cell line that is like at least kind of stable.
So some, it depends. A So these
Clonal though, these are mixed pools?
No, they're not clonal. Okay. They're not clonal. So we just, uh,
do a random integration by antivirus,
which might of course contribute that a large proportion of them just silence
over time.
But this is something we see very commonly in many cell lines to silencing.
So that can be, can take some time until you have a good cus nine line.
Um, and then actual,
So quite a lot of preliminary work before you even get to the screen, then you,
you,
you build the best possible cell line that's gonna be amenable to the screen
being Yeah,
Exactly. So that's important. Yeah, you have to have a good SSS line. Um,
I mean if, you know, if like only like 80% or lower of your sales express cast,
you will pick up all the guides that are in empty sales that don't have cast and
it will cause a lot of background of course. So this needs to be good.
So that's worth spending some time on. But altogether then, uh,
the actual expansion of the sales library transaction, um,
doesn't take that long normally, unless you have sales that grow like really,
really slowly, which also happens sometimes. And then to get like, uh,
240 million or whatever you need to transfuse per,
per replicate can be daunting. How about with normal cells? It's not a big deal.
So this takes maybe two months and, uh,
normally we say to our customers when they come, they can expect data in,
in four to six months. There's also the sequencing of course,
you then need to do the next generation sequencing for which rely on another
facility that does the sequencing. They also have a queue,
so it doesn't necessarily go immediately. Alright. There's
Elements that you have to out your elements of the process you have to outsource
to of the course locally then. Yeah. Okay.
Exactly. So we do everything other than the sequencing. Um, so
Analysis of the sequencing as well. Does it do,
is that something you guys can do or do you work with mathematicians for that
process?
Uh, data analysis we do ourselves, um, with mainly the magic package.
That is a common thing. Uh, there are many others out there,
but we use that one. Um, so we, in the end, there's not that much you can do,
right? I mean, you end up with recounts in control and recounts in treatment.
So you get two, two columns of numbers. Uh,
there's not that much you can do with this, but of course, um,
you can do chin set enrichment analysis then on the, on the data that you get,
et cetera. So we could be better at that,
but we do what we can to help to help our clients.
So you, so you mentioned this other person who, who does the,
the cell and engineering are, are they involved,
like that functional validation?
So if you've got some really exciting hits from your screen,
will that person then go and knock out that cell individually? Sorry,
knock out gene individually the cells to Yeah, I, I see.
Yeah, we, we don't really do it. I mean, you know, the screening, it's,
it's a high throughput method. I I,
I used to say it doesn't actually give you any answers most commonly, but it,
it teaches you to ask the right questions. I, right, uh,
you get a lot of hits that some hits hopefully, that you expected,
other hits that you have no idea why they come up. Um,
so then the validation is the really challenging part. Then the screen is,
uh, relatively straightforward. Um, but then the validation of course,
a validation, make sure that your hits are real,
and of course figuring out the mechanism,
figuring out a story around the whole thing that you find that is way
more time consuming and way more challenging. And that, of course,
we just pass off to our customer again, is, okay, there you go, there your hits.
Um, good luck. Um,
which also contributes to the fact that it can take a very long time from a
screen being performed and the publication coming out.
So there's a often a several year gap.
Yeah, I, I suppose just to comment on that,
quite often you'll see in papers the screen is figure one and then yes,
it follows you. It's probably, you know, a lot more work, uh,
downstream of that. Yeah.
That's how it is. Yeah.
So four to six months is a significant amount of time amount investment
for any investigator. And I assume it's, it's not necessarily cheap either.
Is it quite expensive to run these, uh, these, these, these experiments?
Um, they are, I think a pool screen is actually fairly cheap. Um,
and array screen is considerably more expensive because you, you,
the reagents are one, one time reagents, right? You just use a up. Um,
whereas here everything is, there's no big instrumentation involved. Um,
it's just, it's mainly sary cost because of course it is a lot of work,
but a typical screen that we run, so we, we have a subsidy on that as well. But,
um,
it's probably around 10 to 15,000 pounds.
Oh, okay. More reason I expected. Yeah,
It is not terrible. Uh, it's affordable. Um,
now, sorry, I lost, uh, did you, what, uh, what else did you ask?
I lost your question.
No, I, I was saying obviously a time investment as well, you know, oh,
Time investment. Yeah,
Sure. Or investigator colleagues ever get impatient, you know, do they, uh,
start to ask questions after a couple months, that kind of thing?
Yes, yes, yes. It happens, of course. But, uh, in a way it's,
it's easy for them because it's, as you say, it's normally,
it's the start of a project, right? So it is not, if you make a no cut line,
like you for example, do, uh, then often people also come to us and say, oh, uh,
we were asked for a paper revision to do that. And,
and then of course it's urgent, super urgent. The screen is normally the,
the start of a project, and I think they're bit more patient there. Mm-hmm.
Maybe it's also important to get the expectations, right.
So just tell them how long it's likely gonna take. Yeah,
Absolutely. So I suppose, you know, do you always use the same screen?
You know, because I know you, I've seen some of your papers use like, you know,
the brunello screen screen, which obviously very popular, very well validated,
very well used. But do you have a customized, or do you,
do you generally speak and stick with that same screen?
No, we do a lot of different things. So we also make the libraries ourselves.
Oh, really? Um, depending what people want.
So any custom library that can be smaller or bigger than the standard libraries,
of course, I mean, the bread and butter screen is, is a knockout screen with,
with brunello, this is what most people do.
So we use brunello because we like it. I'm not saying it's the,
necessarily the only good library that's around, but it's a good library. Um,
we also do crisper activation, crisper inhibition, um,
screens. We also have done, uh, screening with a CAST 13 D,
so that's the one that actually targets, uh, R N A instead of, uh, D N A. Well,
Let me ask you on that, on CA 13. So we've been dabbling in CA 13,
and we haven't been very successful with it, not on a screen basis.
We seem to get quite a lot of self death and we think a collateral damage of all
the transcripts. Is that something you've seen as well?
Not at all, actually. Okay.
A mixed literature emerging on this, I think.
Yeah. No, we, we targeted,
so this screen was a relatively small screen for around 150 long non-coding
RNAs. Uh, so I don't know, you know,
they might not be that important for things we have not done really looked.
One should actually do a proper screen, I think,
where you actually hit essential genes as well, and then see how,
how well it works. Um, hits came out of it, um,
as always, I mean, people need to validate it now and see whether,
whether it actually makes sense. Yeah. But it seemed to have worked,
because normally if a screen doesn't work, it's just everything's flat, right?
I mean, you just don't find any hits. Um, if you find hits,
especially in this case where you have several more guides than we had,
like probably 10 guides per transcript,
and this significantly actually pops up. Uh, it's fairly trustworthy,
I think. Yeah. Um, so then we also,
Many, I'm gonna say,
do you get many situations where the screen fails or generally most screens
successful?
Uh, yeah. Fail, it rarely fails. Fails.
What it, what can happen? What,
what changes in different screens is the effect size that you get, i e then the,
the p values, uh,
the significance that statistically significant significance of your, of your,
of your hits. So sometimes it's all a bit blurry,
and then that's what we call on the poor screen. Um,
sometimes can be rescued by redoing the library prep. Uh,
sometimes it happens there in the P C R for the, for the library prep,
but sometimes it's just something else. Um,
but I have to say that the vast majority actually work reasonably well.
At least you can always say, okay, this could have, could have been clearer,
but people always find something. Okay,
there's a sort of confirmation bias there as well, right?
People want to find something.
So that's why I'm then when people try to validate it, say, okay,
show me again what you want to validate so I can look in detail at the data.
Is this really, do I buy this or do I not buy this?
Yeah, I suppose that's where the validation comes in again, isn't it that,
you know,
you basically repeat the experiment in a different way to study the gene in a
bit more detail. And I said, there is a bit of confirmation bias, isn't there?
I suppose that is one of the risks here.
You almost have too much data from what I can tell.
Yeah, it's, uh, it can be a lot of data. It can be, you want,
there's some sweet spot, right? You want,
ideally you want to find like a couple of genes that you were expecting and a
couple that we were not expecting. That's ideal. Mm-hmm. Uh,
if you find only the ones you were expecting anyway, they're disappointed. Um,
if you get 700 hits, um, then they go, oh,
well what are we gonna do now? Uh, so yeah, difficult,
I guess you can run then a follow up,
a smaller follow up screen or something to see in a more,
like followed maybe by single cell transcriptomic readout, for example,
to get more information about the phenotype. And so there are things,
one can narrow it down,
but most people pick the hits they work on, on a,
on a much less scientific, uh, in a much less scientific way.
They just say, okay,
I'm comfortable with working with stuff that's in the nucleus.
I'm uncomfortable with working with stuff that is in the ghi.
So if something from the GHI comes up, she goes, uh, no, uh,
it's not my area of expertise. So we drop that one, we take another one. Right.
So this is more how it works in practice.
Well, I suppose when you, when you publish you, do you publish the, the data,
the entire dataset so that if someone else around the world who is interested in
that goal, you are in the other, so, you know,
for the results in that compartment, they could take that finding forward.
Yeah, ideally. So, um, we have also,
so we are funded from the Swedish government mainly. We also have a mandate to,
to push people into. So big we, we rarely publish ourselves. It's more that,
that our customers publish,
but we should push them into also publishing that the data is,
is published in according to fair principles.
So it'll all be there and it'll be findable and retrievable and, um,
have the metadata present that it can actually be, um,
refound and, and reused.
So what definitely needs to be done is all the original sequencing files are
uploaded to short treat archives so that this, this data is available,
but without the proper metadata, of course this is not very useful,
but there are several databases, uh, for CRISPR screening data that exist.
And we encourage people to, to upload to these
in a good way so that then the data is not lost in a sense. But it's,
it is always hard, you know,
if it comes from different labs done in different ways to make it, uh,
really comparable, it's difficult.
Mm-hmm. Um, I was gonna ask, does,
does everyone at your institute use you guys for functional screening,
or do they sometimes do it themselves in their own laboratories?
Um, screening, I don't know much that people do screening.
So some people have tried and then come to us. Uh,
it, because commonly, you know, the first time you do it,
it's likely gonna fail. Um, you need a practice run,
at least one practice run. I say, okay, if you the second, third time,
I think you're good. It's not that complicated. Yeah. Um, I
Actually really quite nicely leads me onto another question I had in that you as
a newcomer, what would be your, you know, main advice,
obviously expect failure the first time is one bit of advice.
I think you could say that, but, you know, what would you say to someone who's,
you know, started to think about using these techniques in the laboratory?
Um, yeah, assuming the user,
assuming the user published library that you can get ready from,
I think it is not so hard to amplify this. This is pretty easy, otherwise,
library transformation cloning can already be a big problem. Um, okay.
And you get all the guides in.
So this is something where you need to have good protocols for, uh,
for double stranding, the oligo pool and these kind of things. Uh,
if you have a library ready, um,
one advice that I would have is make sure that the way you count cells
actually count cells correctly. Um, because, um,
it seems that many also automatic cell counters,
they seem to overestimate the number of cells by counting some debris in
something.
If you do this several times while you have a screen running and you every time
underestimate by 20, 30%,
you will then eventually pull nick your library and you will lose stuff.
So having a lot of cells, counting them correctly, um,
How, how do you count your cells? Are you the hemo, cytometer,
the old old school? Really? Okay. Old school. Fascinating. You know,
you've got this cutting edge technology and you're using the hemo cytometer.
Do you sell counts?
Yeah, we have, we have, we would have even a fax that could sort cells, uh,
sorry, uh, count cells, but we, no, we rely on the hemo cytometer.
People are used to it. They're good at it. It's quite consistent, so works fine.
Um, yeah. Then if you do screening,
if it's a live dead screen,
so you have a drug screen or you just look for proliferation,
I think it's also still pretty straightforward. Um,
there are good protocols on ing how to prep the libraries and do this kind of
things. So I think this is all kind of All right. Um, what we do a lot, however,
is, uh, uh, screens where you do fax based sort thing,
because in every, any pool screen, right,
you need to separate your phenotypes of interest from each other.
So if your live dead screen is easy, otherwise you need to sort, uh, and again,
in order to not bottleneck, one needs to run, uh,
around a hundred million cells through the facts. Um,
so commonly we sort for two days for one experiment, two full days.
So this is also something to take into account,
especially if you don't have your own sort and rely on a facility needs to be
pre-booked well in advance.
And you have to also tell the poor staff there that they might not be able to go
home at the time they are used to, because it can take even longer.
So, so you use another co facility there in in
No, we have our own sort of You have your own, yeah. Own sort. Yeah.
Otherwise it's, it becomes too difficult.
Yeah. You'd you'd be upsetting all your other colleagues who just wanna go.
Exactly. Exactly. Yeah. 'cause those old screens are run in duplicate,
so it's very important. There's also good advice. I mean,
don't run a screen in only one replicate, um,
because whatever you find, you can never be entirely sure it's correct.
But if you have two replicates,
then you can be sure about things that you would otherwise be unable to call.
Right? If something comes up at position,
rank two in one replicate and rank 150 in the other out of
20,000 genes, then they rank 150. Even if it's maybe not significant,
statistically it still means something if it comes up in post replicates.
So that's really important, I think.
Are your replicates really, generally speaking quite, you know, do you,
you get very similar results outta them? Or is there a little bit of like,
say a bit of a bit of wiggle room there?
Uh, it depends a lot on the screen, the screen type, but um,
they tend to be fairly okay. Um, wouldn't say tight,
but there, there's wiggle room, definitely. Um,
that's why I'm saying you then basically look, okay, uh, you,
you can do an average rank of genes between the two replicates and then see
where they end up. Um, often the, the calculation of p-values and,
and false discovery rates are very, very stringent, often too stringent.
So it's not easy to set the cutoff really, whereas, okay,
this is still worth looking into or is no longer worth looking into.
Yeah, I mean, again, it's down to the research, I suppose,
who receives the data to decide what they're gonna do with it? Yeah,
That's a common question. Where do I put the cutoffs? So, well,
wherever you like, uh, it's a ranked list, um, whether you,
it it seems make seems to make sense for you or not that this is still correct.
And, and that's where the replicates help a lot, right?
'cause otherwise you're totally lost, but if you have two replicates,
then it's easier.
Yeah.
I suppose things that come out as being top ranked are very often things that we
already know. Um, so Right. There's no real follow up for the research to do.
So Yeah.
Very often
I assume people are looking in that, you know, ranks 10, you know,
10 to 30 those kind of genes rather than one to 10. Yeah.
Or if you do screens like we did with the long non-coding RNAs,
of course you stick some protein coding genes in as positive controls and Yeah.
Who already they come up as the top hit. Sure. Um,
good in. But yeah,
So, so you know, your advice would be, uh, you know,
count cells properly use for first time and well published and well protocoled
library, make sure you do things in duplicate, that kind of thing.
Really all very good standard scientific advice in many ways. But yeah,
Yeah. Uh, also read, read the Review by John Dench,
that's called Am I Ready For crispr?
I think we also call it the gospel according to John. Uh,
so that has really, really good advice. It covers it really nicely.
It's quite a couple years open. I I don't think things have changed a lot,
so this is really very, very good advice.
So, you know, speaking of change though,
obviously you say things haven't changed loads,
but do you see change on the horizon?
Are things gonna get very different in the next few years? Uh, what,
what do you anticipate?
Um, yeah, I'm not sure actually. Uh,
the knockout screens, I've, I don't know. I,
the hope is that you could have some, some improvements in terms of, uh,
effect size or have smaller libraries with better guides.
So there can always be better guides, right? It can always improve the,
the prediction of, of guide activity and, and these kind of things. But, um,
there are also things, um,
such as that if you cut with a guide and you re rely on
alga and joining for, for, uh, repair,
that of course you can always get in-frame repair and the problem might not be
disrupted. So there's always some noise in this screen study.
I don't think it's, it's even possible to get rid of,
there will always be to a certain extent, noisy. Um,
what is maybe coming more is rate screens.
Now that is relatively easy to make these guide RNAs, uh,
as synthetic guide RNAs. Um,
is that now any better than was a, an original S RNA screen?
I don't know. Um,
of course it's a different situation if it's a knockout screen,
if it's a crisp inhibition screen, maybe it's not different.
But of course there are things like, uh, sca, um,
CAS 13 D for example, that is even easier to do in,
in an array format or CA 12 a because these have much shorter,
much shorter guide r n a, so this is easier and cheaper to synthesize.
So maybe this is something that's coming more. Um,
and other modalities I think are interesting. So we are looking a lot into,
uh, base editing screens, uh, where you can now,
instead of knocking out an entire gene or activating an entire gene,
you can now actually introduce point mutations. Uh,
so tile and entire gene body with guides introduce point mutations then for
example, C okay, which point mutations are resistant to a drug, uh,
if you know the drug target, right, you would do use this on the drug target.
So this is something we're looking into quite a lot now.
We have not quite succeeded yet. Other people have. Uh, it's a bit tricky,
but I think it's doable. And I think this is a,
is a new frontier where we could get a lot of more information than you can get
from a just whole element gene knockout screen.
Mm. Absolutely. Could suppose its scanner function of the, you know,
or change the function of the
Yeah. Chain function. Exactly. You could also, I mean,
you have to not only think of the drug binding to a target and exactly in the
interface you make mutations. It could also be the steric, uh,
interactions that you can identify what is, you can do epitope mapping,
protein-protein interaction studies, all sorts of things. Uh,
it's not very base editing, it's not very efficient often, but,
uh, I think it's good enough, um, as it is to,
to try to do these kind of screens.
So, uh, you, you know, based it in s p Cass nine all the time,
but you mentioned Cass 12 as well.
Are you using the other casts proteins that cut D N A?
Um, yeah, we have used CASS 12 A for screens. So in,
we use this for multiplexing. So if you want to deliver two guides at once,
you want to hit two genes at once. For example,
if you are interested in synthetic lethality or in generally speaking,
synergistic or buffering interactions between genes works as well with SS nine.
But because of the long trace of SS nine, it's a bit trickier to get it to work.
And with S 12 of a,
it works really nicely also because S 12 of A actually chops its own, uh,
two guide pair into single guides. The problem is,
uh, is not a huge problem and can be, uh, will,
will improve in time is that maybe the guides are not as good. So the,
maybe the algorithms of guide design are not as advanced as for S nine.
So there maybe a,
a higher chance that you have guides in there that actually do not,
do not really work that well. Um,
but we did one screen where we looked at, uh,
synthetically th between all the uase and all ubiquitins,
so is a hundred, I think the screen about a hundred times a hundred, uh,
proteins. And that worked reasonably well, I have to say. So I think it's, uh,
it's a totally workable, um, thing. CAS 12 A,
of course you can always in a, in a precision editing context,
you can still use it if there is no Cass nine pam around, um,
we have used Cass 12 A with good success. Um,
I think it works pretty much the same, it seems.
And obviously one part of this is you, we,
we touched on it before when you mentioned about live dead screening versus
functional assays.
I suppose that assay is really critical in terms of how sensitive it is to
making sure you're separating those population cells according to their
different behaviors.
Yeah, absolutely. I mean, this starts with life dead assays already.
If you do life data asay on a, on a suspension line, for example,
some suspension cells seem to hang out quite a long time,
even though they're dead, but they're kind of intact. If you then harvest,
you harvest also the dead cells with it. And then of course your,
your dynamic range is gone. Um,
more importantly with other screens where like reporter assays where we do fax
based cell sorting, of course, um,
if you do a staining or you,
you do not have necessarily have to do a reporter line,
you can also do staining. So we can also do screens on fixed cells. Um,
then the staining has to be like really well set up. Um,
another important thing with drug screens if you wanna do with drug screen is
that your drug concentration is actually right. Uh,
we do IC 50 and IC 19 normally,
and this is also an important point that this gets tested to get determined
on large culture flasks and not in a 96 will play because it never translates.
Oh, really? Wow. On small play. And then you go like in a T 75 and,
and the IC 50 is like totally different. Um, so that
Something you do, do you, is that your lab testing in the IC 50?
Uh, we tell, we tell our clients to do that. Uh,
They build the assays as well. That kind of function assays too.
Yeah, exactly. So the way we work practically is often that we give the,
the library transducers back to the client who then does their assay
and give us back cell pelles, and then we, um,
we prep the genomic d n a into the wrist.
'cause often they know their assay best, right?
Yeah, exactly.
Trying that.
And sometimes they, are they developing bespoke brand new assays for just,
you know, just for this application? Or is it generally speaking, people say,
we've already got an assay, let's do a screen.
No,
often it's a new assays because you can even consider making a specific report
line for screen. So we, we have projects where we made a T G P,
the responsive reporter line, for example, that has A G F P under A T G F P,
the responsive promoter, and only then you can actually do a,
a proper pulled screen where you can sort out, um, high and low responders.
So that's quite common actually.
So that's where the precision edit goes into the screen, right?
So you have to first do a knockin Yeah.
Make a in line or people wanna compare a knockout line with the wild top lines.
You first make a knockout, then compare these two,
which counts with its own set of difficulties, but okay. Can be done.
Sounds to me, honestly, there's so much, I I,
I could talk to you all day about this and it, it sounds so exciting and I,
I think I'm gonna walk away from this podcast with a renewed enthusiasm to
actually, you know,
start speaking to people locally and follow some of these knockout screens. Uh,
but I think, you know, we've been acting now for just over half an hour.
We probably should, you know, call, call it to a close.
Been absolutely pleasure talking to you today. Really appreciate your time. Um,
and I'm feeling a little less intimidated now about doing these screens,
Uh, not enough in, are you still intimidated enough to not? No,
I don't think so. I, I think, you know, I'm just intimidated thinking about,
you know, my, my, my team probably say, we haven't got time for this.
So I think maybe some conversations about how we, you know,
move some our resources around that kind of thing to make sure we can support,
uh, this, this, this type of experiment. But you know,
there's so much you can do with it,
and we're talking about things that might just scratch the surface and the
things you've described today, how many different ways it can be applied.
It just sounds absolutely fascinating. So thank you so much for your time today,
Ben, Ben Schmear. I apologize again for pronouncing your name really badly. Uh,
it's been brilliant and fascinating talking to you. See you soon.
Thank you so much. It was my pleasure. See you soon.
You've been listening to CRISPR Unedited. To access more thoughts,
help and advice on crispr,
visit bite-size bio.com/crispr-unedited.
Creators and Guests
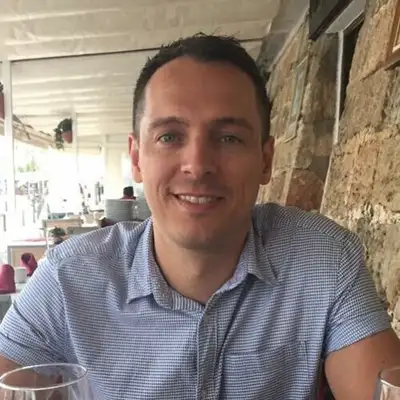
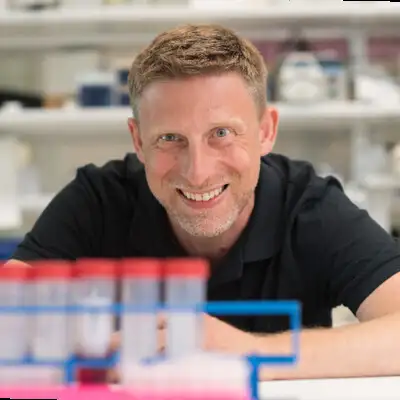
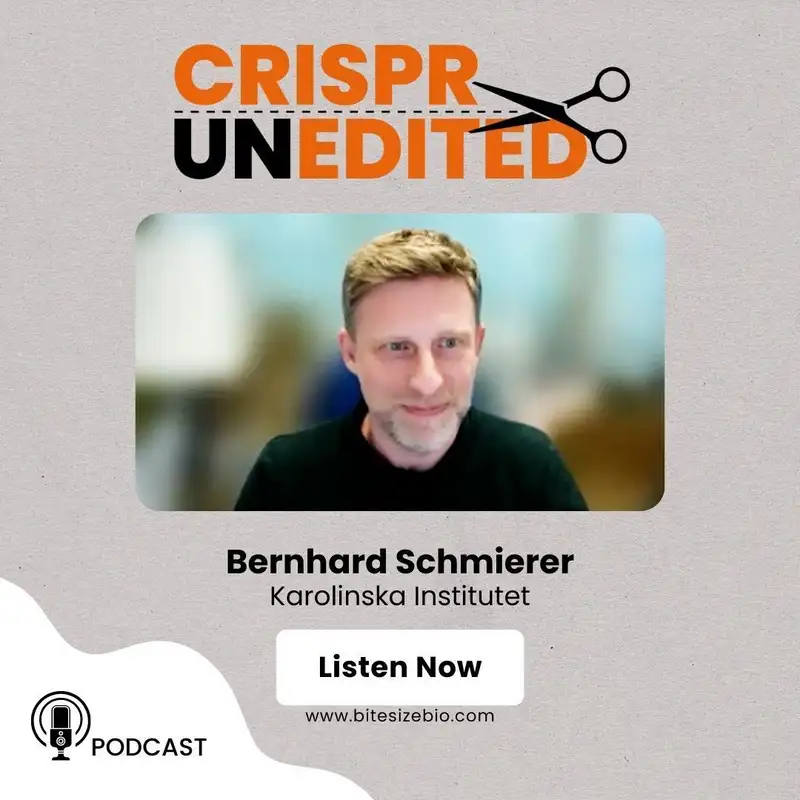